After an earthquake, effective damage assessment is critical to saving lives and planning a rapid and effective response. The assessment needs to accurately and quickly gauge the scale and the extent of the damage and do so without endangering additional lives. A new Japanese artificial intelligence model that uses aerial images from disaster sites is able to do just that.
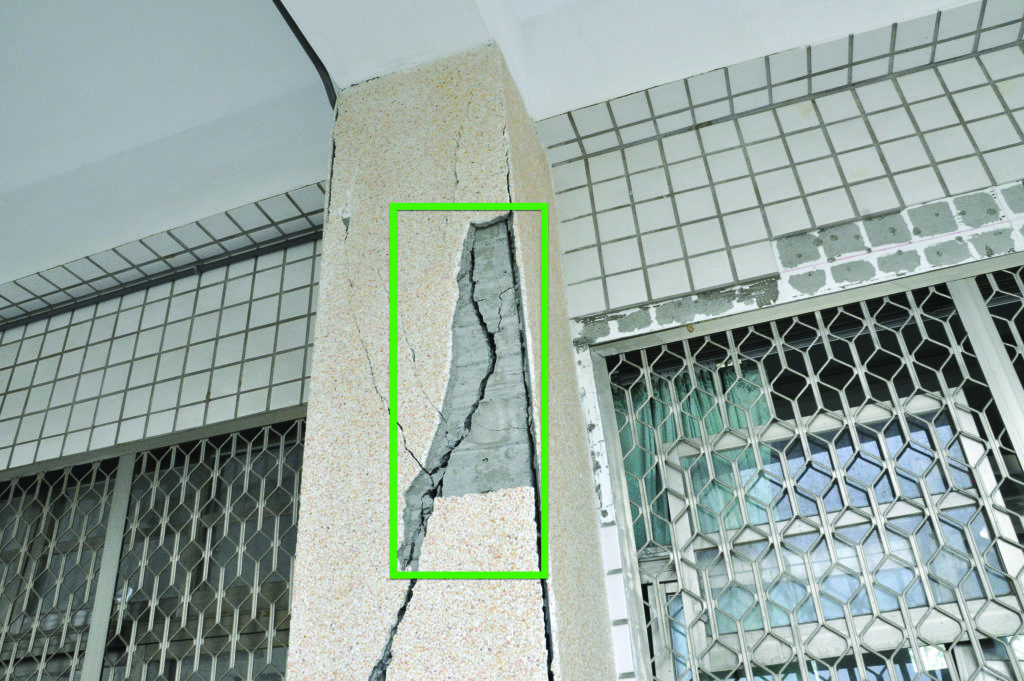
A team of researchers at Hiroshima University in Japan has developed an artificial intelligence system to analyze aerial images taken after earthquakes and quickly evaluate the damage shown so teams can attend to critical areas first. The AI places buildings on a damage scale that ranges from D0 to D6, a scale originally proposed by professors at Hokkaido University in Japan. The scale is used by the Architectural Institute of Japan as the standard damage scale for the country’s timber houses. “Our model identified non-collapsed buildings as D0-D1 damage (and) collapsed as D5-D6 damage,” says lead researcher Hiroyuki Miura, Dr.Eng, an associate professor at Hiroshima University’s Graduate School of Advanced Science and Engineering. However, “it is still difficult to accurately classify the moderate damage levels (D2-D4) from the air,” he adds.
While this scale is specific to Japan, Miura says it is equivalent to the European Macroseismic Scale’s damage level scale (G1-G5) that is used around the world and would be applicable for earthquake damage detection in other countries.
The Hiroshima AI model uses convolutional neural networks, a deep-learning mechanism that achieves image recognition from basic pattern-matching. This type of AI system digests many example cases before it can be applied. In this instance, the AI first trained on pictures from Japan’s 1995 Kobe and 2016 Kumamoto earthquakes.
While assessing damage by comparing before and after images might seem like a more logical approach, Miura points out the many advantages of comparing post-disaster images from multiple incidents. “In emergency responses (taking place) immediately after natural disasters such as large earthquakes, it is time-consuming to prepare and assess pre-event images,” Miura says. “When such pre-event images (are) not available in some affected areas because of the lack of observation, those methods cannot be applied. To overcome this limitation and execute a quick and timely post-disaster response, damage identification only from post-event images would be preferred.”
Shirley Dyke, Ph.D., A.M.ASCE, a professor of mechanical and civil engineering at Purdue University, also works on using AI to compute damage assessment after earthquakes. AI models like hers train on structures for data about columns, beams, interior and exterior images, and more. “Once I’ve developed that structure and trained my (AI) model, I can take any data set from anywhere in the world, put it into that model, and organize the data sets in minutes,” Dyke says.
It is this efficiency that is AI’s biggest advantage. Because it eliminates a lot of grunt work that would otherwise go into sorting and classifying images from the disaster zone, it helps researchers skip to actual analysis faster. “If the data set is prepared, our AI model can classify the damage within a few minutes for 10,000 buildings,” Miura says.
The Hiroshima AI model is not foolproof, but it is 94 percent accurate. “If a building is surrounded by high trees, the damage identification would be difficult because some part or most of the roof is obscured by the vegetation in the aerial image,” Miura says. “For another example, if small features such as garbage or junk are distributed around a building, our method would misclassify the building as damaged because it would be difficult to discriminate such features (from) debris produced from collapsed buildings.” He adds that solar panels present similar challenges. Aerial images also do not always paint a complete picture. “If buildings are collapsed by a soft-story crush (only the first floor is collapsed), our model underestimated the damage because the roofs of those collapsed buildings seemed to be intact in the image,” Miura says.
The workaround is to feed more images to the AI to create an even more robust system. The Hiroshima model trained on approximately 7,000 building “image patches” extracted from 45 aerial photos. “For the AI training process, the image patches were augmented to (form) about 15,000 patches,” Miura says. In the data augmentation process, new image patches are artificially created by horizontally and vertically flipping, rotating, and adding contrast to the original image patches.
While Japan is notorious for its earthquakes — close to 2,000 occur in the nation every year — tsunamis and floods are also common.
The AI model can be useful in these other kinds of natural disasters as well. Miura is training the model with images from the landslide events in Hiroshima prefecture in July 2018 and the flood event in Kumamoto prefecture in July 2020. “To develop a more robust and accurate AI model applicable to various disaster scenarios, not only for earthquakes but also for landslides, typhoons, and flooding, we are updating the AI model by providing these training samples,” Miura says.
The ultimate goal is to make recovery faster and more efficient. “I (hope) that the updated AI model would be used in real disaster situations to provide infrastructure damage information to local municipalities and stakeholders much faster than the traditional methods,” Miura says. “The derived damage information would help actual post-disaster activities such as early stage recovery planning and waste management planning.”
This article first appeared in the December 2020 issue of Civil Engineering.
Errata: The print article originally referred to “conventional neural networks” instead of “convolutional neural networks.” The online version has been updated. We regret the error.
Excellent article and equally excellent application of AI . Thank you